SuperGPQA: Scaling LLM Evaluation across 285 Graduate Disciplines - Exploring the Real Proficiency Boundaries of LLM
Introduction
- Paper Link: https://arxiv.org/pdf/2502.14739
- Dataset: https://huggingface.co/datasets/m-a-p/SuperGPQA
- Project Homepage: https://supergpqa.github.io/
In the rapid tide of artificial intelligence development, accurately assessing the capabilities of AI models has become a key issue in industrial progress. On the journey to explore the boundaries of AI capabilities, researchers have come to deeply recognize the limitations of existing evaluation systems. This has spurred scholars to collaborate with top industry research institutions to jointly break through the established paradigms of AI evaluation. Against this backdrop, the milestone project SuperGPQA has emerged.
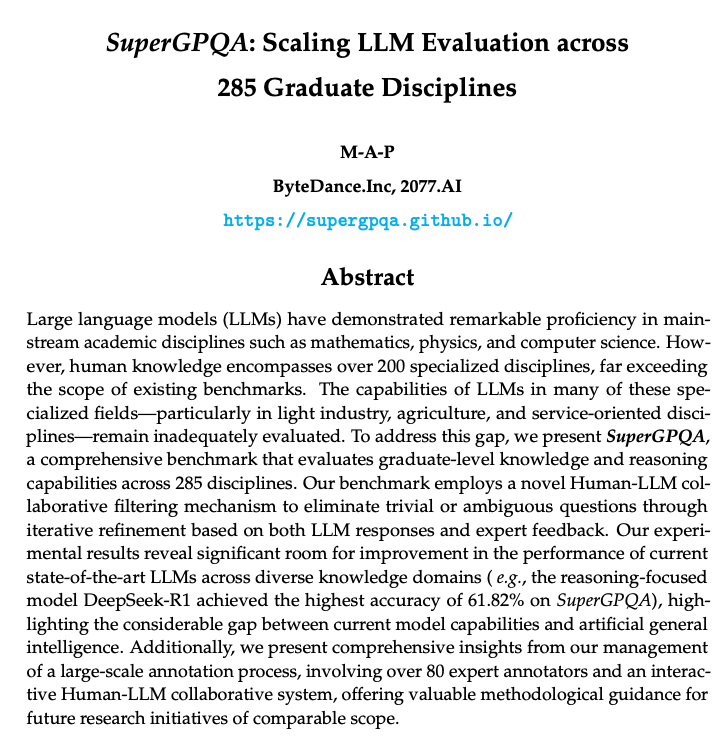
1. Insights on the Frontier: The Real Challenges of AI Evaluation
With large language models like GPT-4 and Claude demonstrating capabilities that approach or even surpass human levels in mainstream academic fields, accurately assessing the true proficiency of AI in a broader range of specialized areas has become an urgent challenge. Existing evaluation benchmarks, such as MMLU and GPQA, suffer from severe imbalances in subject coverage—long-tail disciplines like light industry, agriculture, and service science have a coverage rate of less than 5%, and the discriminative power of evaluation questions is gradually diminishing.
In response to this real challenge, 2077AI, in collaboration with top research institutions, spent six months developing the SuperGPQA project. It has, for the first time, achieved a benchmark for AI evaluation covering 285 graduate-level subjects.
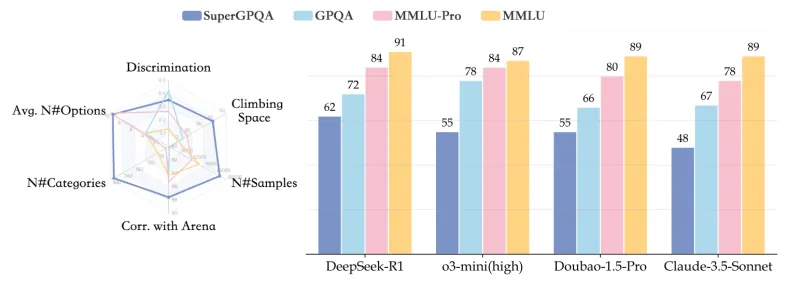
2. Breakthrough Innovation: Restructuring the Evaluation Paradigm
SuperGPQA has achieved breakthrough innovations in both scale and depth. The project has constructed a vast knowledge system comprising 26,529 specialized questions, far exceeding the 448 questions in GPQA and the 12,032 questions in MMLU-Pro. In terms of subject coverage, SuperGPQA spans 13 major categories, 72 first-level disciplines, and 285 second-level disciplines, achieving a comprehensive mapping of the human knowledge system. Each question is equipped with an average of 9.67 options, significantly higher than the traditional four-option format, which greatly increases the challenge of the evaluation. Notably, 42.33% of the selected questions require mathematical calculations or formal reasoning. This design ensures the evaluative distinction and depth of the assessment.
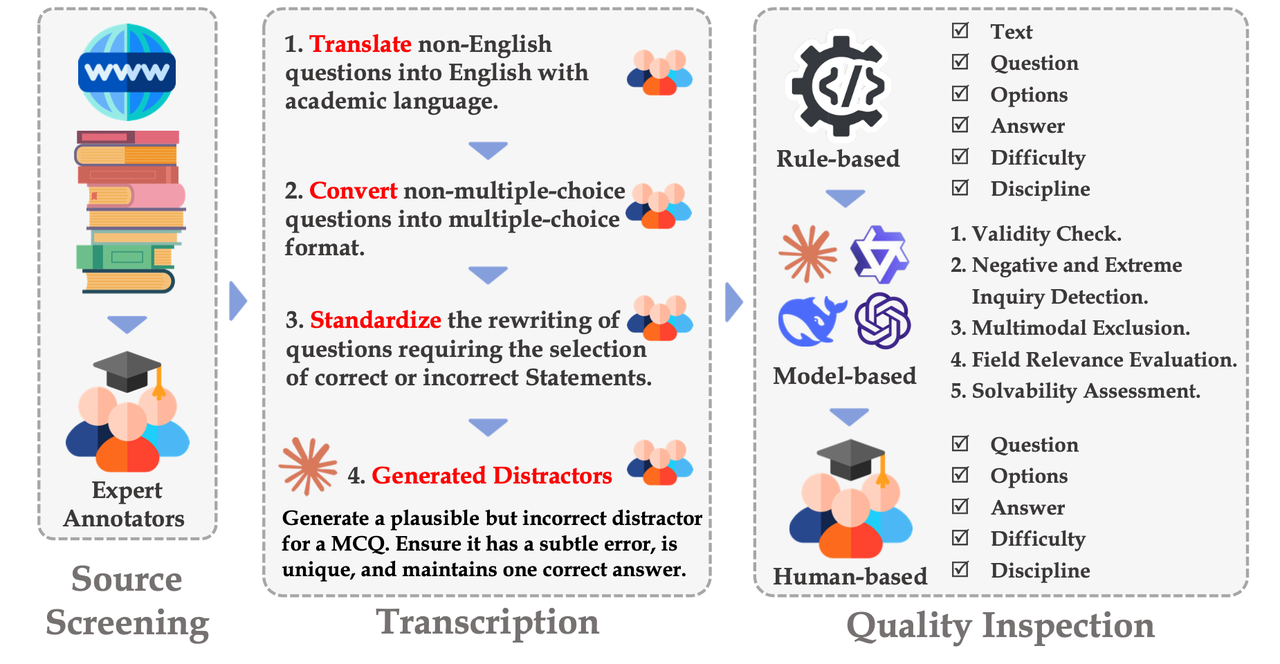
2.1 Technical Highlights: Interdisciplinary Semantic Analysis
Through t-SNE visualization analysis, the research team discovered that SuperGPQA exhibits a unique interdisciplinary clustering pattern in the semantic space. Questions from engineering and science demonstrate high semantic similarity, while those from the humanities maintain their distinct knowledge centers. The clustering of different disciplines achieves a complete mapping of the diverse human knowledge system. This distribution characteristic also validates the scientific nature and comprehensiveness of the evaluation dataset.
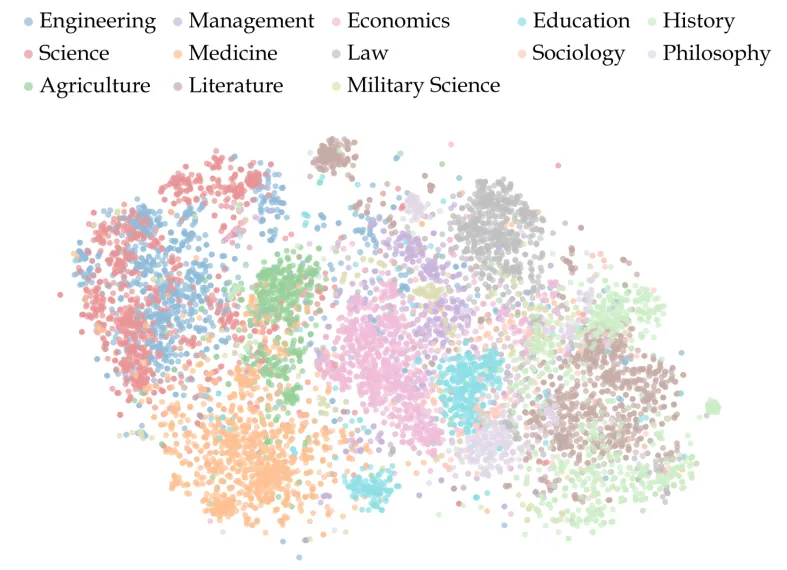
2.2 Methodological Innovation: Three-Stage Quality Control
To ensure the reliability of the evaluation, the project team has designed a rigorous three-stage quality control mechanism.
In the source screening phase, the SuperGPQA team abandoned traditional crowdsourcing methods and instead selected original questions from textbooks and authoritative materials by a team of experts.
In the standardization transcription phase, a professional team normalized the academic language and unified the format of all questions, ensuring that the average length of each question was maintained at 58.42 characters and guaranteeing the consistency and comparability of the options.
In the quality inspection phase, the research team integrated automated rule checks, cross-validation by multiple models, and in-depth expert reviews to build a robust quality assurance system.
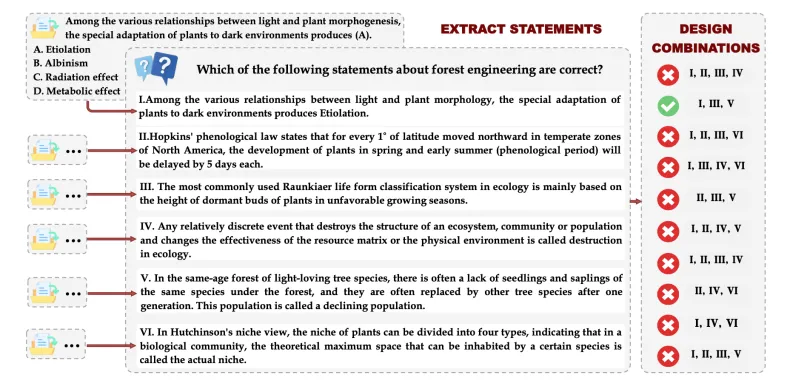
3. Key Findings: Revealing the Boundaries of AI Capabilities
Through systematic evaluation of 51 mainstream models, the research team has made a series of important discoveries.
Under the evaluation criteria of SuperGPQA, even the best-performing DeepSeek-R1 model only achieved an accuracy rate of 61.82% in answering interdisciplinary questions. This result clearly reveals the significant gap that exists between current AI and general artificial intelligence. Experimental data indicates that instruction fine-tuning has a significant positive impact on model performance. For example, the accuracy rate of the instruction fine-tuned version of DeepSeek-V3 (47.40%) is far higher than that of its base version (32.14%).
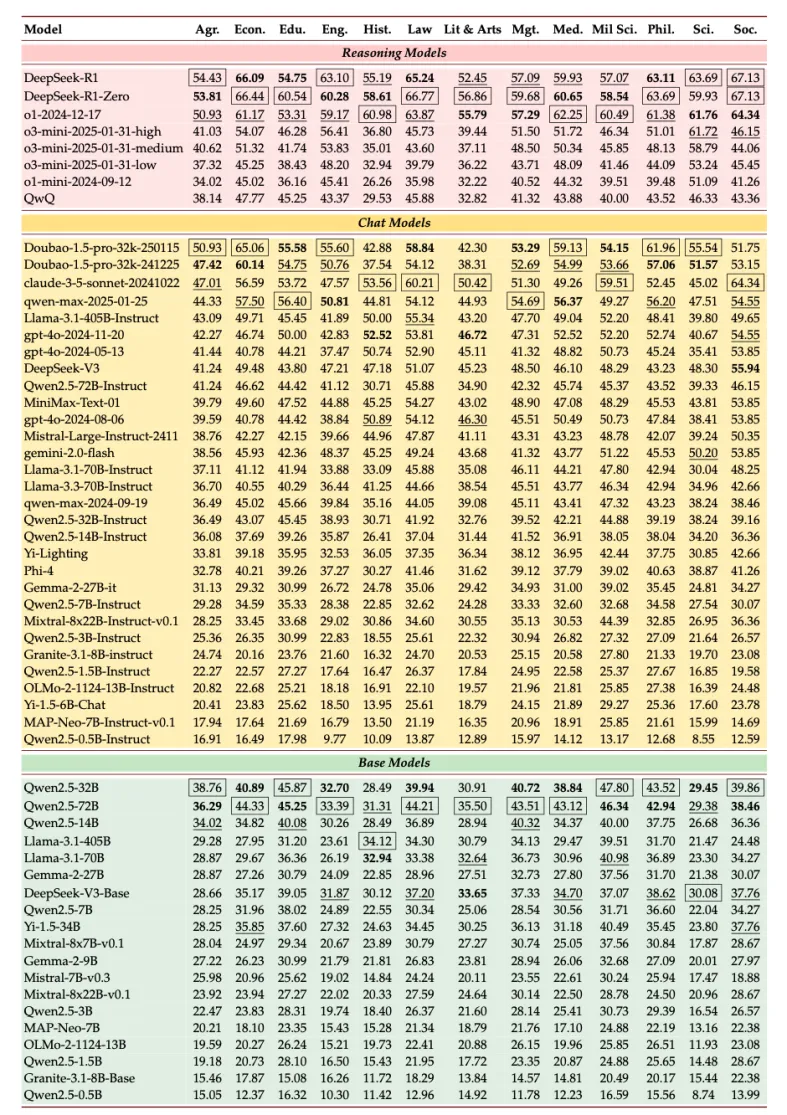
During the in-depth analysis, the research team observed a clear correlation between model scale and performance equilibrium. DeepSeek-R1 demonstrated stable performance across questions of varying difficulty levels, with an accuracy rate of 63.59% on easy questions, 63.63% on medium-difficulty questions, and 56.87% on difficult ones. Additionally, the performance improvement brought about by model version iteration was also significant. For example, the accuracy rate of the GPT-4o series steadily increased from 39.76% to 44.40% with each version update.
4. Future Outlook
The open-source release of SuperGPQA not only fills an important gap in the field of AI evaluation but also pioneers a new research paradigm. This breakthrough provides the academic and industrial communities with a reliable "compass," guiding the direction of AI technology development. As a core participant in the SuperGPQA project, 2077AI, together with the project team, has jointly planned the future development direction of the evaluation system. The research team will continue to expand the dimensions of evaluation, introduce more refined assessment criteria in specialized fields, develop dynamic difficulty adjustment mechanisms, and build cross-lingual evaluation capabilities. On the methodological front, the project team is committed to optimizing human-machine collaborative evaluation mechanisms, developing adaptive question generation technologies, and establishing a more detailed capability classification system. Meanwhile, the SuperGPQA team will also vigorously promote the open-source sharing of evaluation standards, establish a global collaborative research network, and foster in-depth integration of industry, academia, and research.