PIN Dataset: A Unified Paradigm for Multimodal Learning
2077AI Foundation is proud to introduce our new project, the PIN Multimodal Document Dataset
. This initiative emerges from our in-depth analysis of the current developmental bottlenecks in multimodal large models, aiming to catalyze exponential growth in multimodal AI through innovative data formatting.
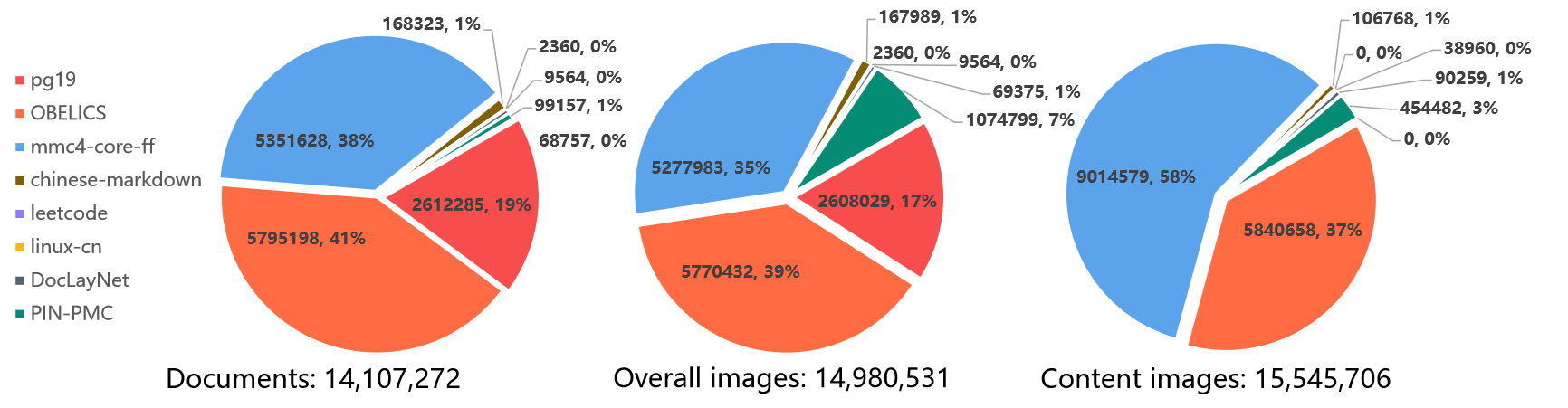
General statisitcs of our PIN-14M dataset.
PIN: A Unifying Paradigm for Multimodal Learning
The core philosophy behind the PIN project is to establish a data format that unifies multimodal learning processes and patterns. We've observed that while the text domain has solidified a "text-in, text-out" paradigm, the multimodal realm still lacks a cohesive, efficient training methodology. The PIN format is designed to bridge this gap, offering a more intuitive approach to blending image and text data training.
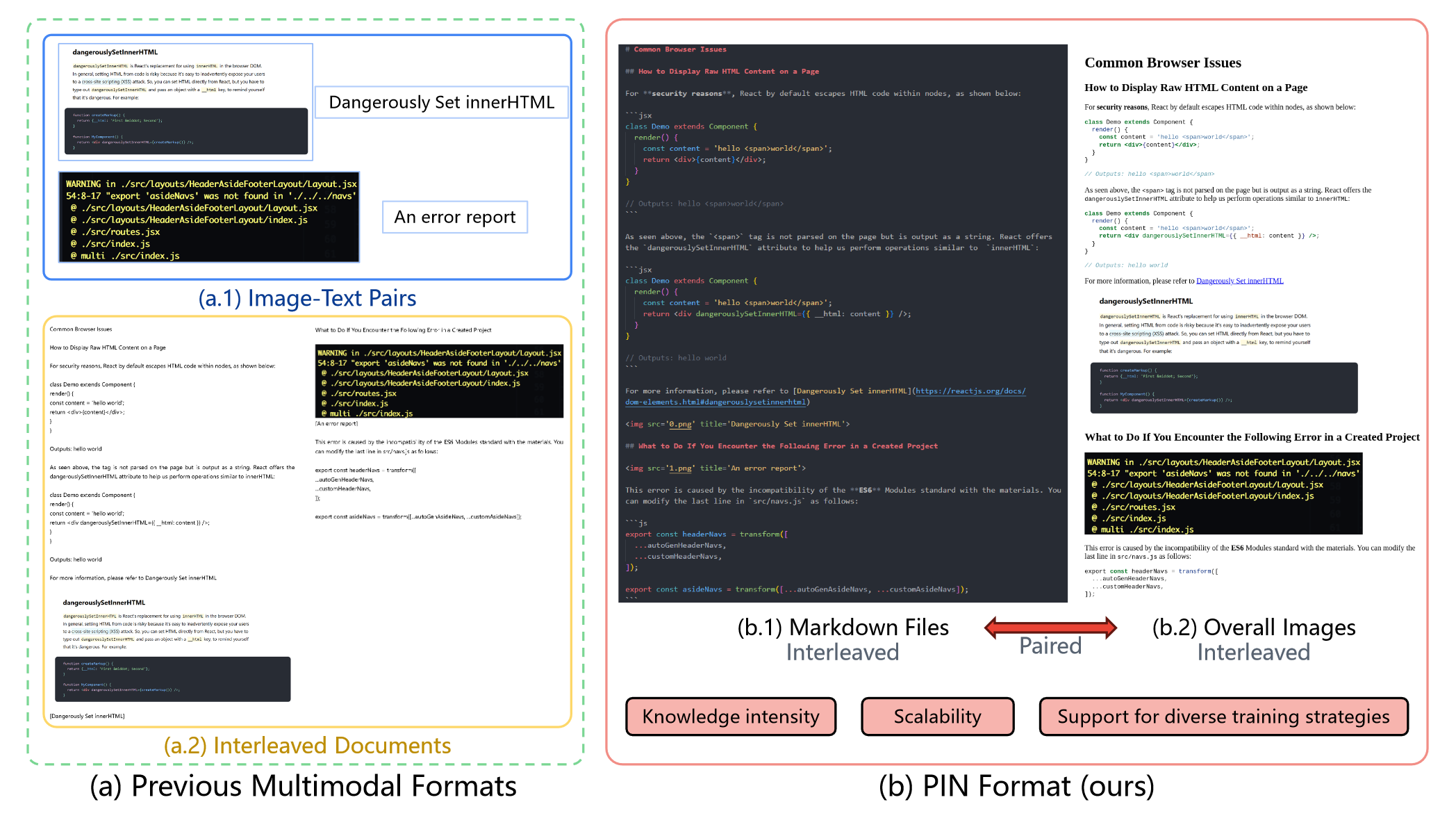
Comparative analysis of traditional multimodal formats versus the proposed PIN format. The PIN format preserves rich knowledge attributes (e.g., bolding, highlighting, code), supports semantic interplay between images and text in markdown files, and enhances knowledge representation through an overall image.
Innovative Data Architecture
PIN introduces a unique "holistic pairing with interwoven content" structure:
- Holistic Pairing: Each sample comprises a Markdown file coupled with a corresponding comprehensive image.
- Interwoven Content: The Markdown file encapsulates text and embedded images that are intricately linked to the comprehensive image.
This architecture addresses the limitations of current image-text pair formats, such as overly concise captions or low relevance issues.
Markdown: The Optimal Knowledge Vehicle
- Facilitates detailed articulation of knowledge attributes
- Supports multi-modal embedding (text, images, code, etc.)
- Preserves document structural integrity
Comprehensive Image: Dense Visual Knowledge Representation
- Encapsulates rich visual data, including page layouts and design elements
- Excels in representing complex visual constructs like code blocks and diagrams
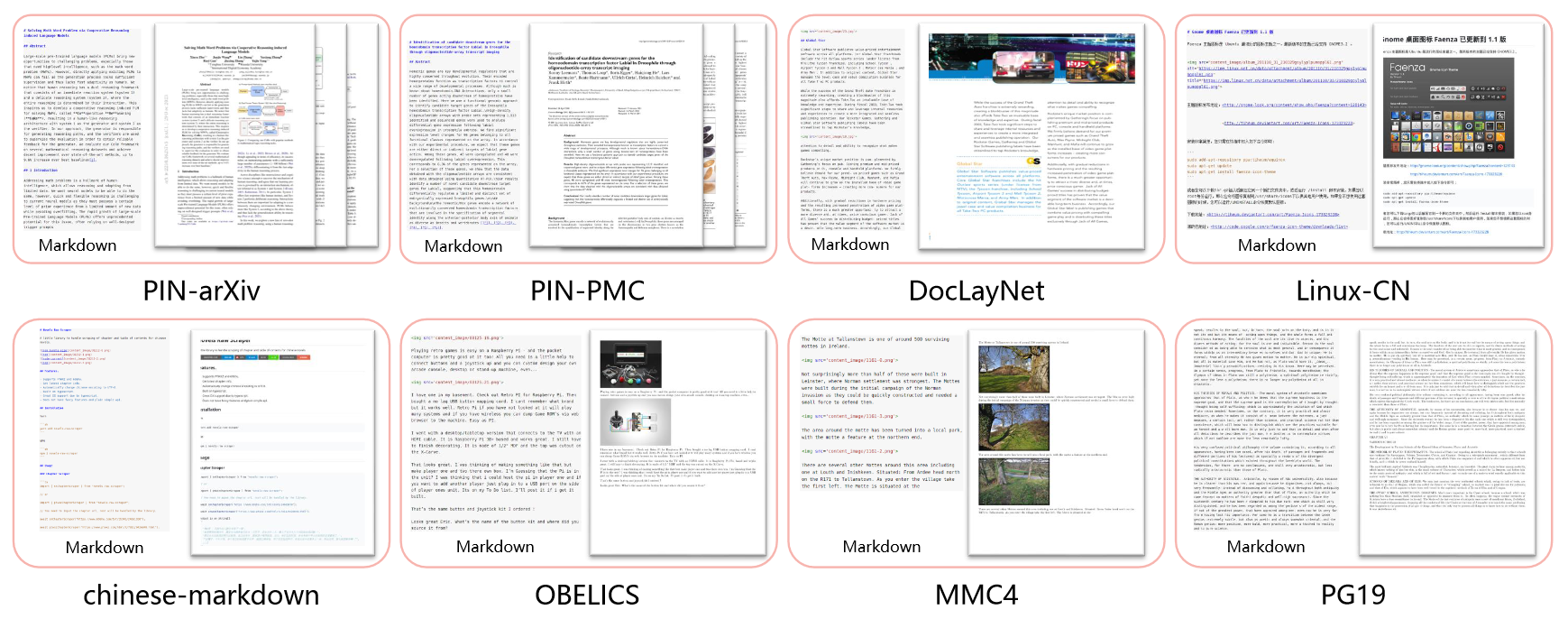
Samples from various subsets of the PIN-14M dataset. For each subset, one entry is extracted, showcasing both its markdown file section and the corresponding overall image.
Pioneering a Unified Format for the Future
PIN is not merely a novel data format; it's the genesis of a unified standard for multimodal data. We envision PIN as a blueprint for future dataset creation, encompassing:
- Conceptual Framework: Detailed exposition of the dataset's design philosophy and objectives
- Procedural Transparency: Full disclosure of data processing workflows
- Quality Metrics: Integration of reliable quality indicators within the data
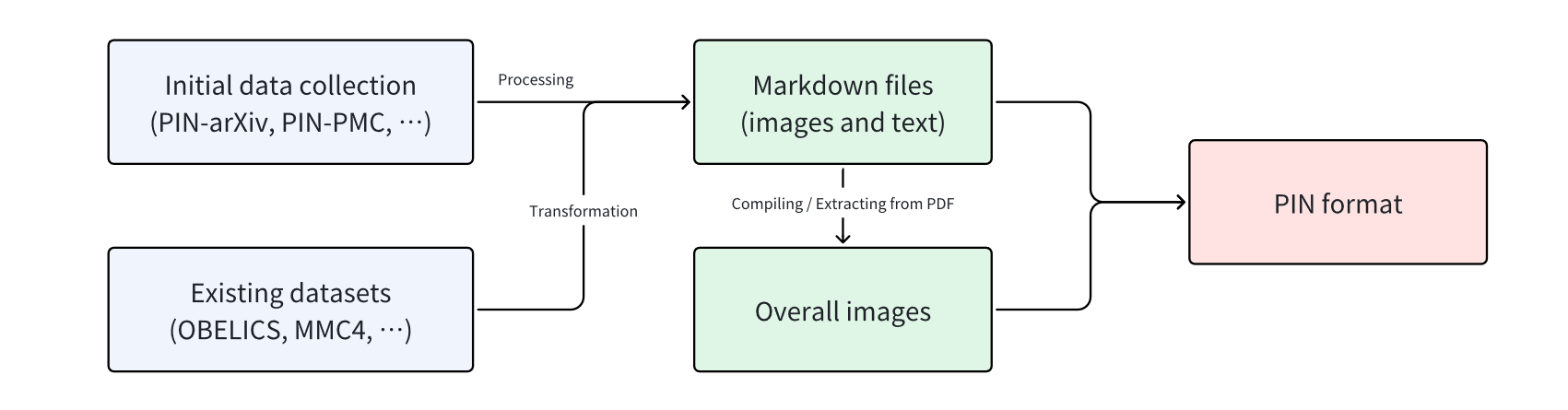
The overview of our process workflow.
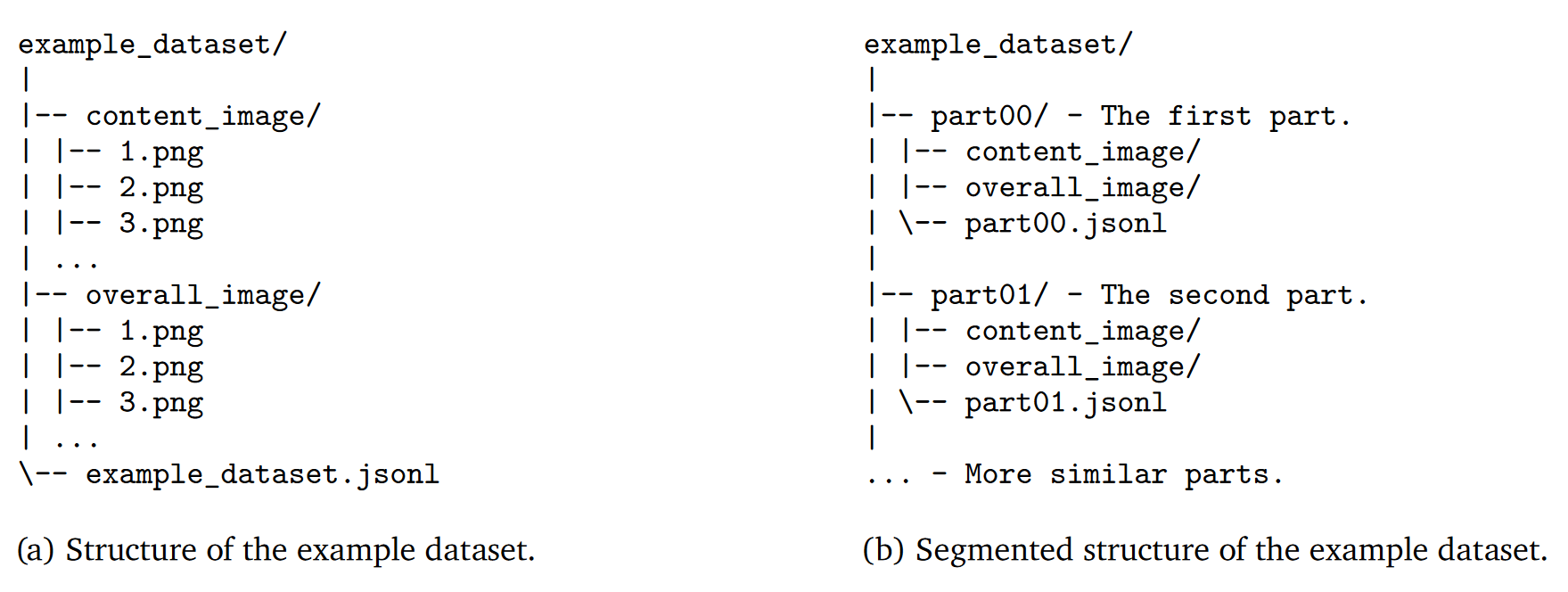
The file tree structure of an example dataset in PIN format.
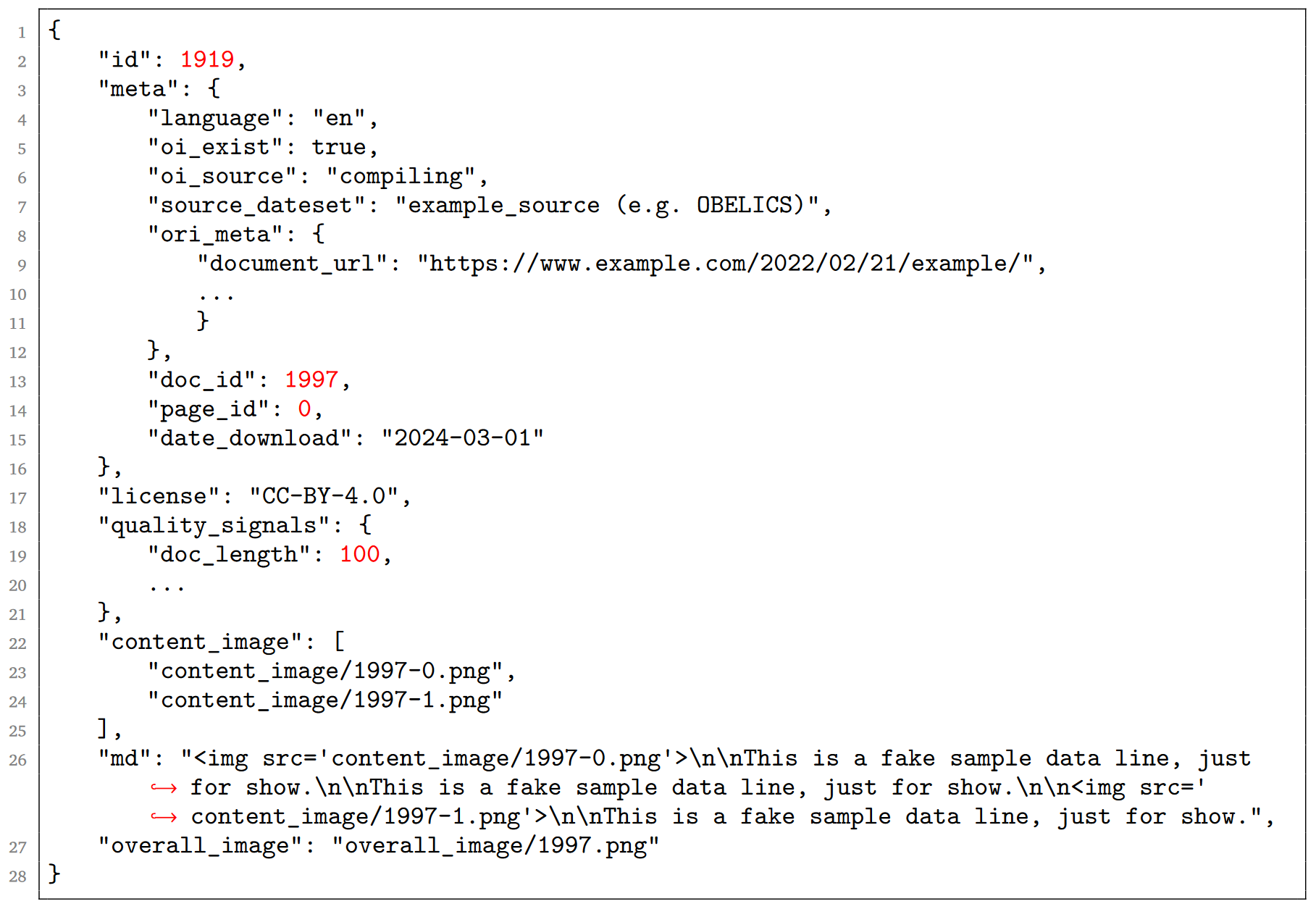
An example data sample of JSONL files.
Conclusion
We have already released initial data sets. Our next iteration aims to expand the data scale to 100M while open-sourcing training models to foster community research.
Although comprehensive training experiments are pending due to time and resource constraints, the PIN format opens avenues for several innovative training methodologies, including:
- Markdown-based prediction of comprehensive images
- Extraction of structured textual knowledge from comprehensive images
- Multi-task learning: Concurrent training in text comprehension, image understanding, and cross-modal reasoning
The PIN project represents a significant stride towards our vision of a more intuitive, unified, and robust learning paradigm in multimodal AI. By addressing the fundamental challenge of data formatting, we believe PIN will pave the way for transformative advancements in multimodal large models. While there are still many areas to explore, we are confident that collaborative efforts within the community will accelerate progress, ultimately leading to a quantum leap in multimodal AI capabilities.
We invite the global community of researchers and developers to join us in exploring the full potential of the PIN format and pushing the boundaries of multimodal AI. Together, let's shape the future of this exciting new era in artificial intelligence.
Commitment to Open Source
Adhering to the principles of openness and sharing, we have not only open-sourced all the data but also made public the code for all processing pipelines, including filtering, deduplication, document conversion, and all other procedures. This allows researchers and developers to delve into every step of data processing and even adjust and optimize according to their needs.
Join Us in Shaping the Future of AI
The launch of the Matrix Dataset marks a new milestone in AI pre-training data. We cordially invite AI researchers and developers worldwide to join us in leveraging this rich resource, pushing the boundaries of AI technology, and collectively shaping the future of AI.